Changing The Game – Liquid Neural Networks – And Tennis
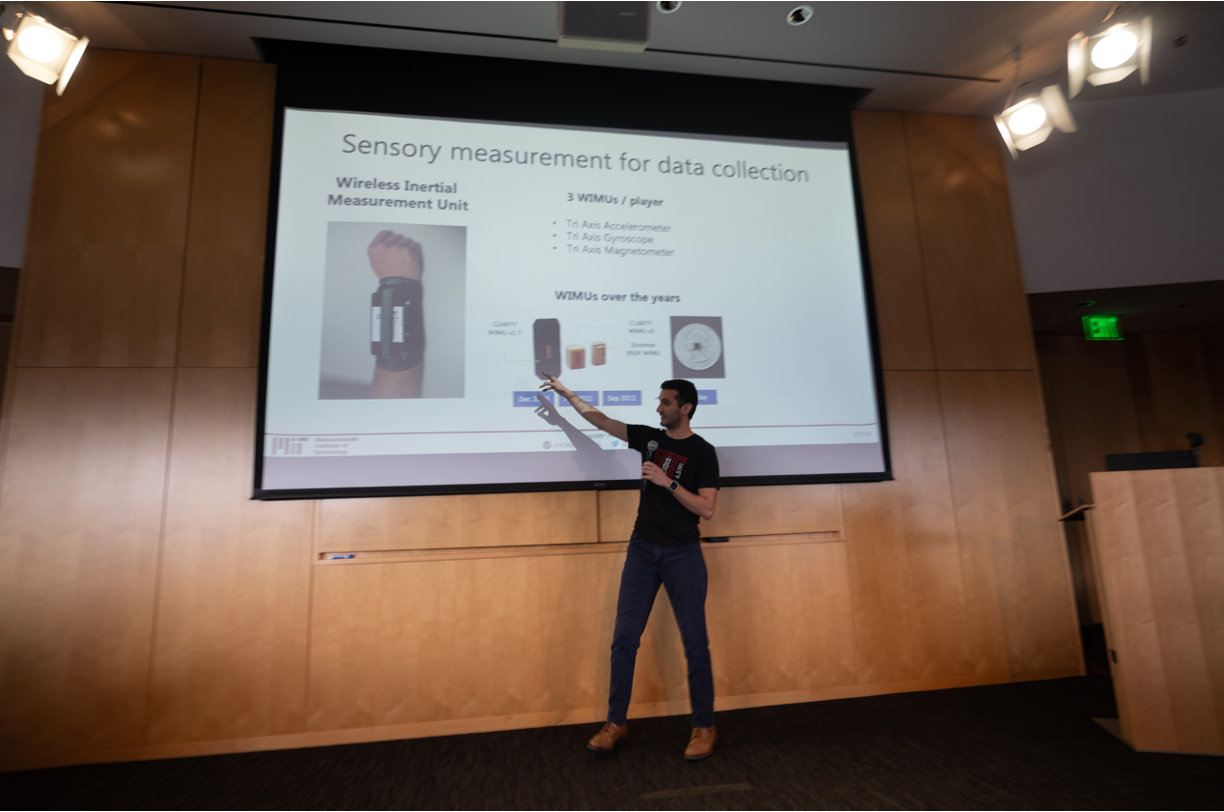
[ad_1]
These new kinds of networks are revolutionizing how we think about AI
AI economic impact and Artificial intelligence disruptive technology that is significantly … [+]
getty
In so many ways, we’re headed into a very exciting era of innovation – artificial intelligence and machine learning are worlds further ahead than they were just a couple of years ago. (That really should be evident to almost everyone, but, it sets the stage.)
One of the easiest examples for people to see is the convergence of new tools like ChatGPT, Sora and everything else that’s springing up today, often without a lot of foreshadowing.
But those of us with a front row seat are seeing something else, too – new forms of neural nets that do a lot more with a smaller and more compact build…
In a prior post, I talked about liquid neural networks and closed-form continuous-time models.
Alexander Amini presenting in MIT Class
John Werner
I wanted to go back to this, because we got some interesting insights from MIT AI scientist Alex Amini at a recent MIT class that we’re putting on weekly. We got some interesting thoughts on what’s actually happening behind the building of these systems. Amini is also a cofounder of a company called LiquidAI, that is pioneering the above-mentioned systems.
To illustrate how he initially approached AI, Amini started with two fundamental examples of projects he has been engaged in, not now, but in the past.
The first one is a fascinating look at his earlier life as he grew through adolescence – Amini chronicled how he moved to Ireland at the age of 14, and became involved in two of his biggest hobbies – coding and tennis.
How did they go together?
Well, he took a complex system, a system of observation, you might say, (I’m referring to watching the game) and then … he changed the game, quite literally!
Amini talked about how sensor systems of the day were usually located courtside – they viewed the action from a spectator’s seat.
But he thought it would be better, in some ways, to put the cameras on the players. And that led him to some further thoughts on how this kind of time-series information gets captured; for one thing, the body’s anatomy represents a unique data set.
“Just the location of one joint can tell us a lot about how the rest of the body is configured,” he said, “especially if you’re in a constrained environment, like playing a sport, right? If … one arm is moving in a certain way, there’s a very finite set of different joint angles that the rest of the body (will) follow.”
He also spoke to some of the broader use cases:
“What we were really creating was a personal refinement system, for behaviors and for motions … (the subject didn’t) necessarily even need to be an athlete,” he said. “So then we later on, I realized this … much broader vision of the company, and then scaled it to other domains, like rehabilitation, medical rehabilitation, where patients are learning to walk again.”
Alexander Amini presenting in MIT Class
John Werner
His other prime example was from his PhD work, much later, some years ago, in looking at neural net progress – after, as he pointed out, he had gathered more mathematical acumen and experience in engineering.
This other insight has to do with the rules-based systems, and the issue of constraints.
“AI doesn’t do well with constraints in general,” he said.
Responding to a question from the audience, he talked about how text, for example, is hard to generate in visuals.
“We only made that solution after we found the problem,” he said, discussing ways to move past these obstacles to more and more vibrant AI capabilities.
Again, the solution is changing the game – looking at new models and new ways of doing things.
As I mentioned before, CfCs are small networks based on studying the nervous systems of certain small organisms. They use artificial synapse models to represent the data transfer between neurons.
Amini also discussed how people view these as continuous processing nodes of a system, and how that allows them to build something much more compact and efficient, shrinking the size of the neural network from maybe a thousand neurons, down to, say, 19…
Alexander Amini presenting in MIT Class
John Werner
In terms of solving some of the constraints previously mentioned, he called for a kind of “auditor” to sit on top of the model, and watch it, and figure out if it is making a mistake.
Alexander Amini presenting in MIT Class
John Werner
Alexander Amini presenting in MIT Class
John Werner
Alexander Amini presenting in MIT Class
John Werner
Alexander Amini presenting in MIT Class
John Werner
Alexander Amini presenting in MIT Class
John Werner
In some of the past lectures that we’ve had where people talk about the neural network’s focus, they’ve explained that it may be processing vision differently than in a traditional network.
These new liquid AI neurons have a range of practical applications – our MIT CSAIL lab Director Daniela Rus can tell you all about their potential for self-driving vehicles, for example.
You can read about more about that in future posts, but these forays into innovation are something I think is central to our sense of where AI is headed right now.
(Full Disclosure: I am a advisor for LiquidAI.)
[ad_2]
Source link